【 Research Outline 】
The popular applications in the sports market have been using human movement data acquired by small sensors. The conventional biomechanics and sports science field define human movement by mathematical average model derived from featured points of the movement. Parameters selected from the model are applied to the final model provision. However, if the model can be found without applying such parameters, breakthrough can be provided to the new movement analysis method that accelerates health management and skill analysis. This research project applies a machine learning approach to human movement bigdata such as movies and sensor data recorded from human movement and tries to find a new method that automatically build a skill model. Read More
1. Research backgrounds
(1) Applications to sports and healthcare using motion data are in fashion
We can find systems that analyze motion data from sensors such as accelerometer and gyro. For example, Nike + that judges jogging and miCoach of Adidas are typical, and OMRON has released a posture meter that judges walking skill. In this way, movement data can be used for self-evaluation of training and judgment of skill level, and is widely used by people who enjoy sports.
(2) The conventional motion prediction / skill judgment method applies individual parameters to the average model of motion.
However, since the systems using the sensor as described above add the parameters for individuals to the average model established in the fields such as biomechanics and motor learning, it is necessary to standardize the parameters. There is a difficulty such as an increase in error in medium- to long-term movement prediction.
(3)The method of predicting movement in the near future in the medium to long term lacks a decisive hit
Akitomo Kaneko’s “Tradition of Techniques”, Koichi Furukawa’s “Physical Knowledge”, Kumiko Ikuta’s “Waza Language”, etc. have tried to find breakthrough. The motion prediction system is not found yet. The problem is that the judgment of “movement in the near future” is left to the user’s self-judgment.
2. Research objective
In this research, as shown in Fig. 1, as a new approach to the conventional method of customizing the average model, we discovered the mechanism of skill from big data of movements in which information on all movements is latent, and “in the near future on a year-month basis”. Is it possible to predict “movement”? We challenge to find the answer. In other words,
(1) Is it possible to specify the skill level?
(2) Is it possible to predict movements for skill acquisition?
The solution to these two questions is derived from data analysis using intelligent information processing, and we aim to develop a self-growth training system whose accuracy improves.
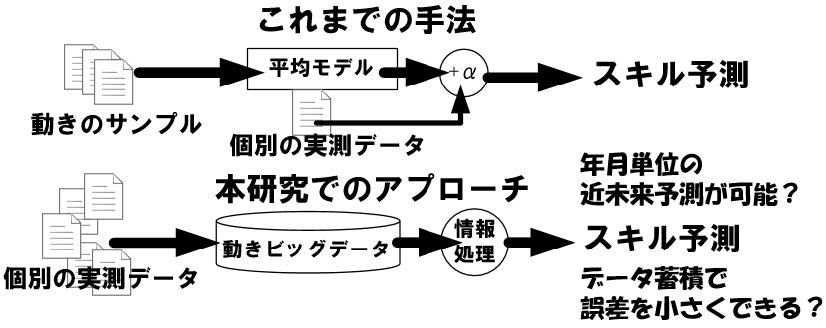
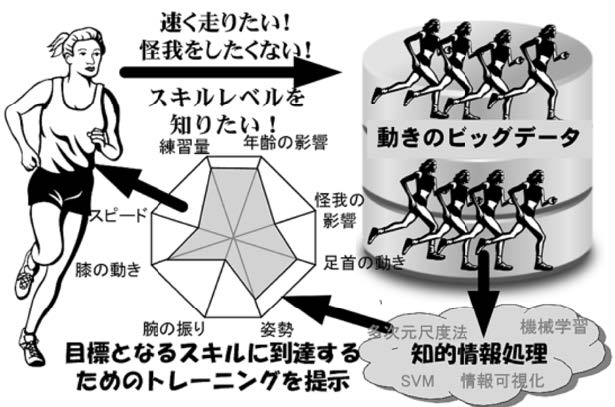
3. Research outcomes
(1)Development of skill grouping method
In this research, we have developed a mechanism “skill grouping” that derives the skill “score” using “movement big data” that collects a lot of movement data. We have developed a system that guides users to approach their ideal movements and a technical platform that provides the services. The movement data can be collected during daily life by even a smartphone with a motion sensor. It will be a familiar technology and can collect a lot of motion data. Therefore, by using motion big data in a timeline, the method can absorb the bias for statistical values.
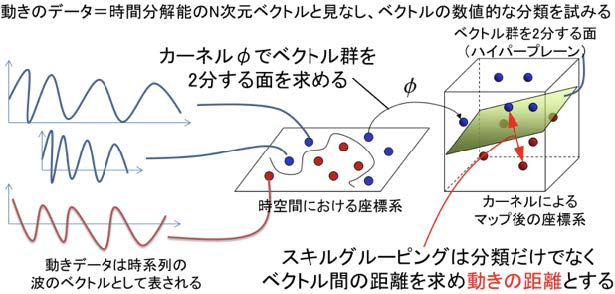
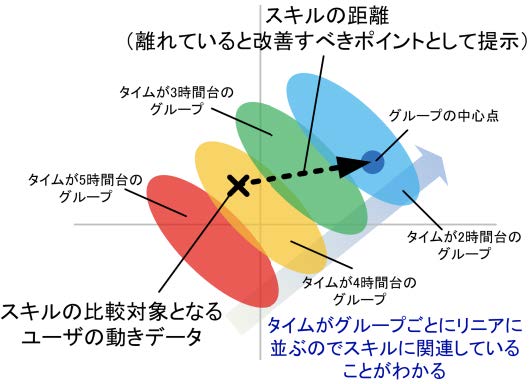
In the skill grouping method, when the user makes a comparison with an expert, we wondered if it would be possible to find the mathematical distance of the motion data. Therefore, as shown in Fig. 2, the “distance of movement” is derived by a calculation for determining the distance between the classified data by the kernel method, which divides the similarity of data into two. At this time, as shown in Fig. 3, if a group is created with attributes related to skills such as marathon time, the movements of parts related to skills are distributed linearly. Skill grouping not only automatically discovers parts related to such skills, but also the movement data of related parts is displayed as an index for skill acquisition, and after sending movement data from a terminal such as a smartphone. , By processing the above processing on the server and returning the score, it is possible to provide a new service that automatically provides coaching.
2)Demonstration experiment of skill analysis
As a proof experiment, with the cooperation of Mizuno Corporation, we conducted to analyze the skills for improving the marathon time by skill grouping and find the effect of the method. As shown in Fig. 4, the running motion is obtained from the images taken by the high-speed camera of the three-dimensional positions of the markers attached to six places on the body. When the three-dimensional position of each marker is applied to skill grouping, we found the important points in the elbows, knees, and ankles as the keys to increase the marathon time. The skill distances of each runner’s movements linearly change with the time. From the movement big data of these three points, the degree of influence on the time (a numerical value that becomes 100 when the time is far from the good group.) is shown on the radar chart as shown in Fig. 5 for the skill. In the form of the degree of influence, we demonstrated the possibility of constructing a service that shows the points to be seen when approaching the movement of the target.
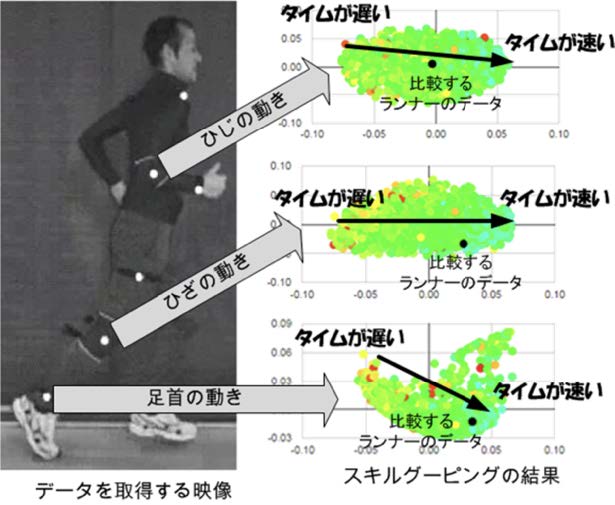
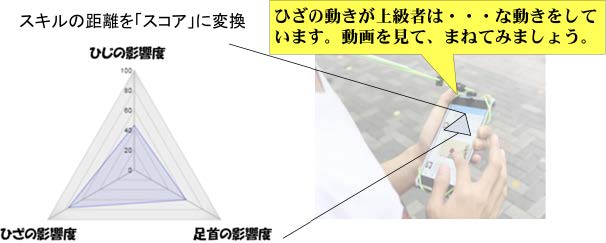
(3)Demonstration experiment for conditioning application
In the ski skill certification (so-called badge test), the judges evaluate the skills by the perspectives. We wondered if it would be possible to automate the evaluation with a computer, and applied skill grouping to the parallel turns of skis to develop a ski training system. As shown in Fig. 6, during the demonstration experiment, three beginners and three experts were asked to carry their smartphones on their backs, and their accelerometers were used to collect the movements of parallel turns and apply the data to skill grouping. In the graph of Figure 6, the eight attempts of each experiment participant are plotted as the same line for each person. The different distributions of experts and beginners represent the skill differences, but the eight trials of each skiers are plotted in the narrow range for the experts and the large range for beginners. In other words, it turns out that experts can used to follow the snow surface in the same way in any slope, but beginners do not. Therefore, it was demonstrated that it is possible to provide a conditioning service to check whether the skill is improved by using it as a material that can judge whether the skier performs always the same movement or not.
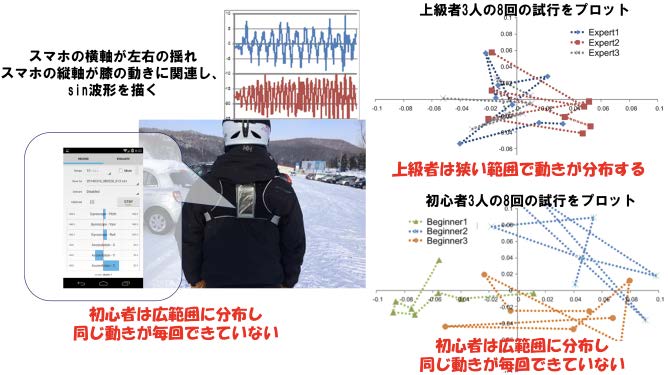
(4)Demonstration experiment for motion analysis of tool differences
Furthermore, in a baseball bat swing, as shown in Fig. 7, an accelerometer / gyro sensor was attached to the bottom of the bat, and the movement of tee batting was acquired from professional and amateur players. The skill grouping was applied to the dataset. At this time, the types of bats were metal and wooden, and the same player was asked to swing them, and the results were compared.
The labels A4 and A5 in the graph in Figure 7 are IDs that identify players. They are plotted in different positions when different bats are used. In other words, the difference of tools induces a difference in skill, which means that the batting motion changes. From this experiment, it is demonstrated that a tool fitting service that automatically determines the fit to the user’s body can be developed by quantifying the difference between tools by the skill grouping.
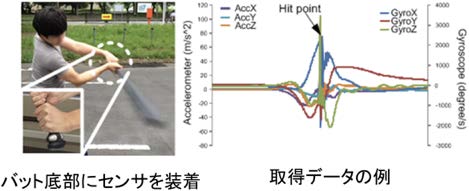
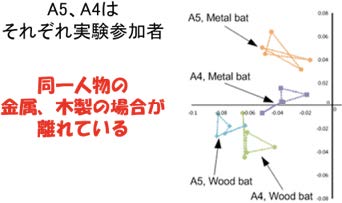
Conference Proceedings
- Shinichi Yamagiwa, Yoshinobu Kawahara, Noriyuki Tabuchi, Yoshinobu Watanabe, Takeshi Naruo. Skill grouping method: Mining and clustering skill differences from body movement BigData, 2015 IEEE International Conference on Big Data (Big Data), , (2015-10-29), DOI:10.1109/bigdata.2015.7364049